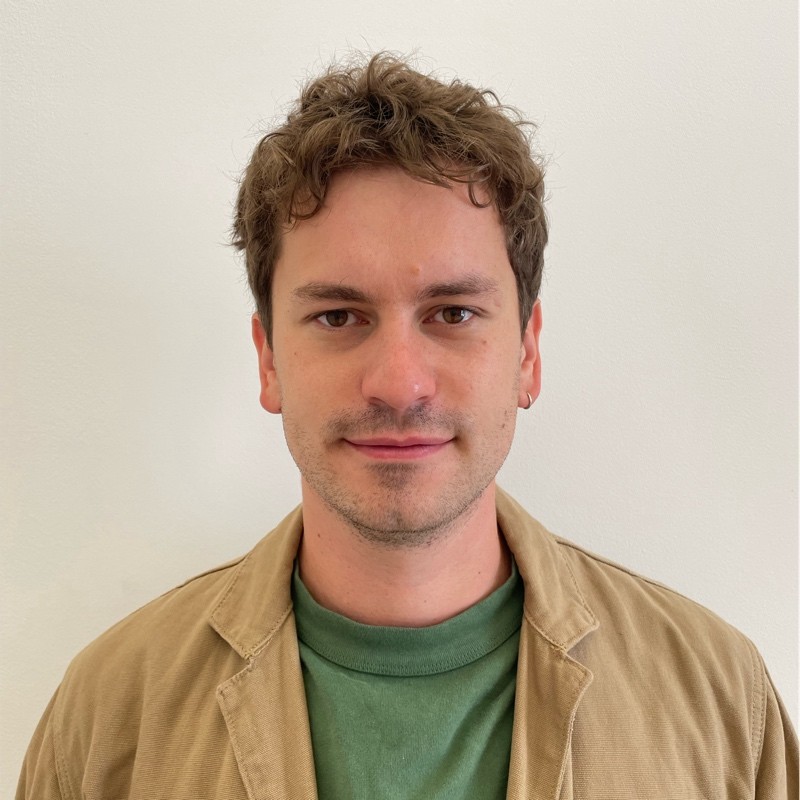
Round 2
Will Grimond
Nationality
British
Career-level
Early-Career
Host institution
Complexity Science Hub
Austria
Residency project
Quantifying algorithmic inequalities: what is ‘fair’ when it comes to AI?
The study of algorithmic fairness is not a new area of research, but it has often been approached from the perspective of singular disciplines: such as philosophy, anthropology or mathematics. The Complexity Science Hub is attempting to marry various approaches to deal with the question of what constitutes ‘fair’ in the context of automated decision-making, and for the first time quantify how bias perpetuates itself among networks along various social characteristics.
The team is currently focused on how social networks tend to mirror the marginalisation of groups in the outside world. To better understand this they are taking a highly experimental approach – reverse engineering and testing the algorithms that govern social networks to see if they can avoid sidelining minority groups. These researchers have already published studies on how bias might be mitigated in AI systems reliant on human feedback, and how political echo-chambers form online.
Read MoreI would also be looking to real-world examples of algorithmic decision- making, and unpacking how these systems work for a lay audience. This would mean sourcing case studies, and perhaps even interviewees, directly from the research these scientists are carrying out. Using this I hope to place the organisation’s research within the wider context of recent developments in machine learning, (inter-)governmental regulation and common ethical concerns with these technologies.